Session 2: The Data Revolution and the Urban Environment: Unlocking the Power of Machine Learning
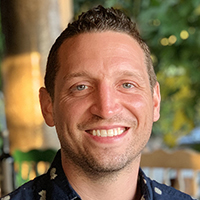
Thomas Manjarres
Energy Engineer
Franklin Energy, Chicago
Franklin Energy, the program implementation contractor for the Peoples Gas and North Shore Gas energy efficiency program, has been working in collaboration with the Chicago Art Institute on an innovative initiative. Pioneering an energy saving technology that has implications for how cities can both save energy and reduce their carbon footprints, machine learning (ML) technology can leverage a building’s existing data to minimize energy consumption, operating costs, and downtime. The machine learning technology deployed at the Art Institute of Chicago is currently being used to optimize HVAC cycles and yielding both electricity and natural gas savings. Results from this project show that combined gas and electricity consumption can be reduced by over 16 percent, generating nearly $200,000 in annual utility bill savings (with commensurate greenhouse gas reduction benefits).
On the surface, machine learning may appear to be overkill for this control problem. Because HVAC can be easily modeled during the design phase, HVAC systems are perceived to be well-defined and well-behaved. This is true for a single piece of equipment from a single manufacturer operating at design conditions but does not remain true over time as equipment ages, new components are added, and the system is asked to operate outside the original design criteria. The ad-hoc equipment in operation today combine to form noisy systems that are often non-linear and poorly behaved. Therefore, machine learning is a tool uniquely suited to solve this control problem. Machine learning models get to know the system as-installed, tracking the inevitable performance degradations over time, and incorporating that into the control strategy so that all equipment always operates at its maximum attainable efficiency.